-
Blog
The search is on for ‘next generation’ specialized AI chips
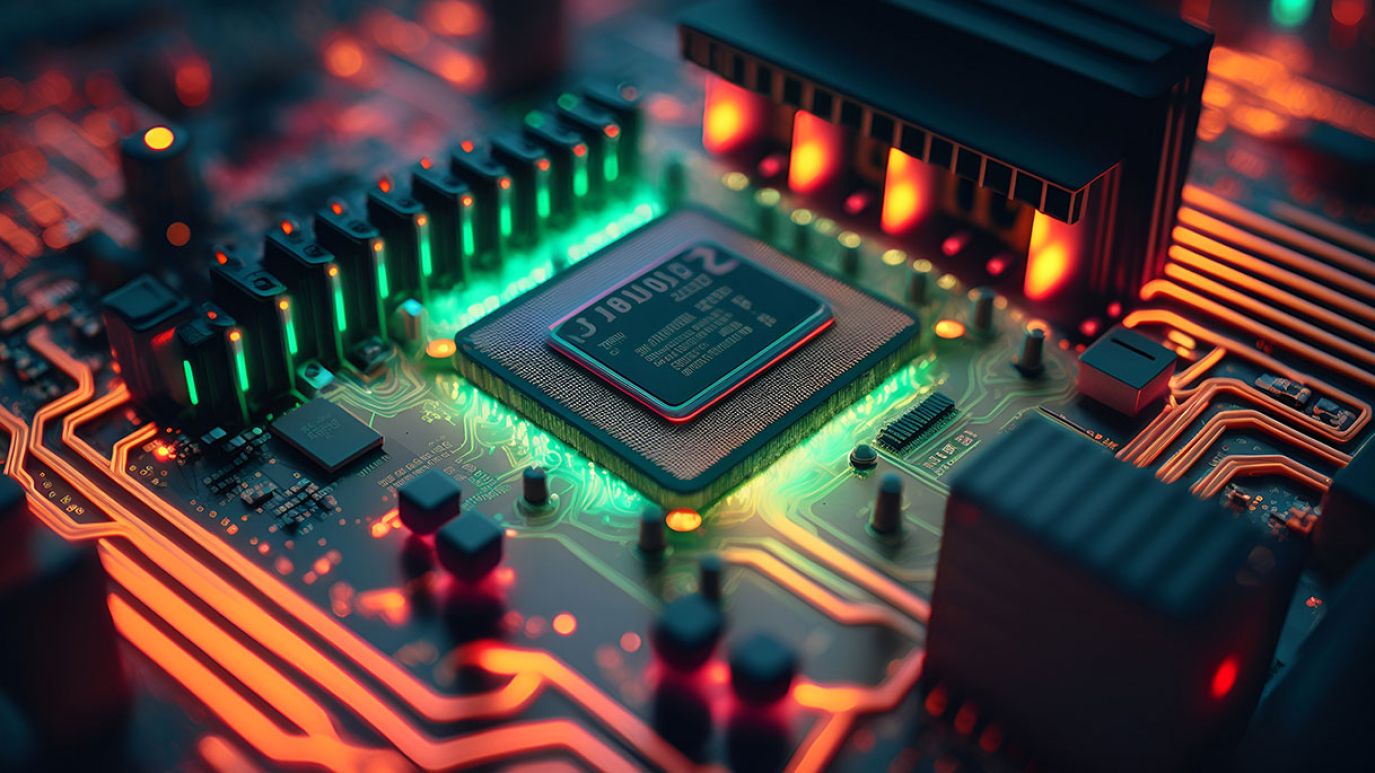
As an extension of the on-going semiconductor trade wars between the West and China, the search is now on for next-gen AI chips that offer much greater processing power and speed, and significantly lower energy consumption.
The advent of AI will revolutionize many industries, but there is a growing concern over its environmental impact. To train an AI model, such as Open AI’s ChatGTP, requires huge amounts of processing power, which in turn uses large amounts of energy and consumes significant volumes of local freshwater supplies for cooling purposes.
Traditional CPUs are not suited to the demands of AI computations, so chip companies such as Nvidia and Google have developed GPUs – graphical processing units – to meet those demands. As AI is embedded into an increasing number of devices and for new use cases a step-change is required in terms of increased processing power and reduced energy and water consumption.
It is widely reported that OpenAI spent around $4.6 million running 9,200 GPUs for two weeks to train its most recent GPT-3 AI model, which reinforces the need for cheaper, faster, more efficient AI processors. So, the search for new types of chips is thoroughly underway.
Photonic AI chip breakthrough in China
In April 2024, researchers from Tsinghua University in Beijing and the Beijing National Research Center for Information Science and Technology released a groundbreaking research publication showcasing a new kind of neural network chip named Taichi, which uses photons, instead of electrons, to perform advanced AI tasks. It is 1,000 times more energy efficient than Nvidia’s H100.
Using neural network layouts on chips. This method is not particularly new, but the use of photons instead of electrons aims to increase computing speed (i.e., performing at the speed of light) and is a technique in its infancy. The new Taichi research demonstrates that photonic chips can now perform real-world tasks.
There are two types of photonic chips already – diffraction-based neural nets (which rely on the scattering of light beams for computation but cannot be reconfigured) and interference-based neural nets (which send multiple beams through a mesh of channels and rely on how these beams interfere but use a lot of energy). Taichi uses a hybrid of both models to eliminates the disadvantages of each.
The chip was able to perform tasks more quickly than existing GPUs and with a similar accuracy. Previous photonic chips possessed thousands of parameters (connections), but Taichi offers 13.96 million parameters.
Intel chooses neuromorphic route for advanced AI chips
Further highlighting the need for new AI-focused chips, Intel recently announced that it had deployed the largest-ever neuromorphic system, called Hala Point, at Sandia National Laboratories, which is run by the U.S. Department of Energy’s National Nuclear Security Administration. It will be used to support research into human brain-inspired AI models.
Neuromorphic computing is a chip design that functions more like the human brain, with the basic premise that the more neurons on a chip, the more powerful it is. It uses neuroscience-based principles, such as asynchronous processing, event-based spiking neural networks, integrated memory and computing, sparse, and continuously changing connections to achieve orders-of-magnitude increases in energy efficiency and performance.
The neurons communicate directly with one another, rather than traveling via the chip’s onboard memory, significantly reducing energy consumption. According to Intel, the system can provide up to 16 petabytes per second (or 16,000 terabytes per second (Tb/s)) of memory bandwidth – for comparison Nvidia’s H100 offers 3.35 TB/s. Hala Point can process more than 380 trillion eight-bit synaptic operations per second, and more than 240 trillion neuron operations per second.
While the system is still considered ‘bleeding-edge’, Intel hopes that neuromorphic computing will ultimately rival Nvidia’s GPUs, which currently dominate AI computation and processing systems. Some of the use cases outed include real-time continuous learning for scientific problem-solving, running complex logistics systems, smart city infrastructure management, and large language model training.
The complex supply chain for advanced AI chips is mainly based in North America and Europe
Likewise, in April, semiconductor startup EnCharge AI announced that its partnership with Princeton University has received an $18.6 million grant from the US's Defense Advanced Research Projects Agency (DARPA). The aim is to solve the problem of inferring – the process a trained machine learning model uses to draw conclusions from new data – to better handle Generative AI applications on devices.
While much of this is leading-edge, it comes at a time when AI is set to be deployed across the board, and the search for faster, more energy-efficient AI-focused chips is strategically important. The U.S. government – through regulations such as Federal Artificial Intelligence Environmental Impacts Act 2024, which was passed on 1 February 2024 – is seeking to limit the diffusion of AI-related technologies, notably to China.
Such leading-edge AI chips will confer an advantage, given that much of the current research and complex supply chain for AI chips resides in the U.S. and Western allies, which provides the opportunity for export controls.
Pamir considers it essential for the U.S. to adopt policies that safeguard advanced AI chip intellectual property and maintain appropriate control over its diffusion, given the huge potential of AI for multiple applications, including network control and management capabilities, and military applications.
Pamir has decades of experience working with North American organizations to determine risk exposure and to ensure compliance with regional regulations. To find out more, contact us today.
China’s 5G influence in developing economies
China’s Belt and Road Initiative and its digital counterpart, the Digital Silk Road, threaten to displace US telecom and tech companies in developing economies in Africa, Latin America and the Middle East. How can US operators and network providers stand up to the challenge?